Project Background
Scientific Questions:
The key scientific question is what can be seen, detected and/or identified under degraded environments, such as during fog, snowstorms, sandstorms, poor lighting, etc. Can we exploit properties of the electromagnetic field including polarization, coherence, spectrum, etc. to gain more information about a scene? What types of photonic (hardware) and software (machine learning) co-optimizations are necessary? In our laboratory, we explore various aspects of such questions via simulations and experiments. In this project, you will have the opportunity to interact with other lab members, and gain experience in both simulations and experiments. We are motivated not only by such fundamental questions, but also by the subsequent applications including autonomous ground, sea, air and space-based vehicles as well as fundamental questions in biological microscopic process (such as the impact of temperature on neural processes). We also collaborate widely across disciplines (Biology, Physics, Neuroscience, Math) and across Universities (Tampere, MIT, RIT, UCF, UT Austin, Harvard).
Student Role
Basic Approach & General Tasks:
The selected student(s) will perform simulations using custom built software as well as perform experiments to measure the performance of various optical systems (cameras and microscopes) under various illumination conditions. If time permits, outdoor measurements will also be conducted. Advances in machine learning algorithms are also of interest to our lab. Please see these references for more information.
Flat Lenses for Imaging:
• O. Kigner, et al, Opt. Lett 16 4069-4071 (2021).
• M. Meem, et al, Opt. Exp. 29(13) 20715-20723 (2021)
Machine-learning for imaging:
• U. Akpinar, et al, IEEE Transactions on Image Processing 30, 3307-3320 (2021).
• S. Nelson & R. Menon, arXiv:2011.05132 [eess.IV] (2020).
• S. Nelson, et al, OSA Continuum 3(9) 2423-2428 (2020)
Machine-learning for microscopy:
•R. Guo, et al, Opt. Exp. 28(22) 32342-32348 (2020).
•R. Guo, et al, Opt. Lett. 45(7) 2111-2114 (2020).
Student Learning Outcomes and Benefits
Learn and apply machine learning to solve important real-world problems. You will interact with PhD students and other undergraduate researchers to learn about optics, cameras and imaging in general. You also have the opportunity to learn experimental techniques, data analysis and of course, machine learning!
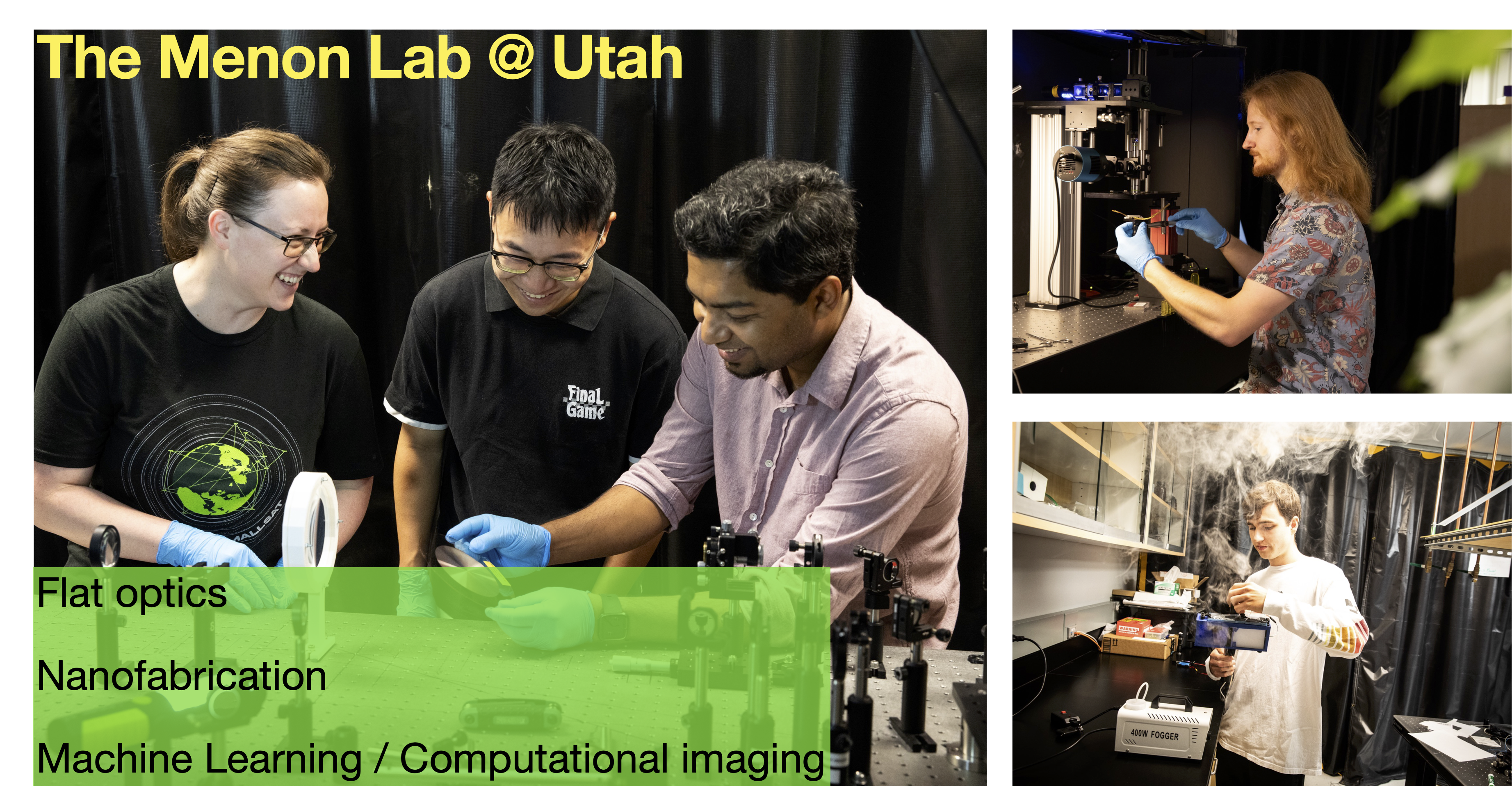
Rajesh Menon
A brief summary of the current research projects in our Group is given below. A common theme is the interaction of light and matter at the nanoscale, and applications enabled by these and related novel photonic phenomena.
Computational Imaging
We combine our expertise in nano- and micro-fabrication with advanced computational algorithms to enable ultra-high sensitivity color photography, spectroscopy, and hyper-spectral and lighfield imaging as well as imaging using a cannula inside the mouse brain.
Digital Metamaterials
We apply computational techniques to design and fabricate new photonic devices based upon digital metamaterials. By combining our understanding of fabrication with photonic simulation techniques, we have demonstrated the world’s smallest polarization-beamsplitter, highest efficiency polarizer, sharpest waveguide turns, etc. We demonstrate with both free-space and integrated photonic devices.
Overcoming the far-field diffraction limit
The far-field diffraction limit is a fundamental barrier in optics, familiar to most as the key limitation of optical microscopy. We are pursuing several technologies that can circumvent this fundamental limitation and enable optical nanopatterning at high speed. The ability to sculpt nanostructures over large areas with exquisite fidelity will enable numerous applications and unleash the power of nanotechnology.
Absorbance Modulation Optical Lithography (AMOL)
By placing a photochromic layer atop a conventional photoresist and exposing with a ring-shaped spot at one color and a bright spot at another, we can achieve patterning of features beyond the far-field diffraction limit.
Patterning via Optical Saturable Transformations (POST)
In POST, the recording medium is comprised of photo-switchable molecules that undergo reversible transitions between two isomeric forms A and B. By incorporating an additional irreversible transformation, we can show that deep sub-wavelength nanopatterning may be achieved at low light intensities and with simple optical systems.
Ultra-high efficiency photovoltaics via Diffractive Spectrum Separation
By separating the solar spectrum into smaller bands and absorbing these with optimized photovoltaic devices, it is possible to achieve sunlight-to-electricity conversion efficiencies of 50% or more with relatively inexpensive materials. We have developed novel microstructured optics that can very efficiently perform this separation and also concentrate sunlight onto the different solar cells.
Optimized Nanophotonics for efficient ultra-thin-film photovoltaics
Light-trapping nanostructures atop ultra-thin photovoltaic devices have the potential to increase light absorption and significantly enhance efficiency of such devices. We have developed algorithms for designing such nanostructures that take into account fabrication constraints. Furthermore, we also explore novel device geometries that have the potential for even higher photovoltaic efficiencies.
3D tracking of surgical instruments
Tracking a surgical tool during non-invasive surgery is an expensive and challenging problem. In this undergraduate research project, we are working with engineers at GE Healthcare to utilize the popular gaming console, the Microsoft Kinect for accurate, robust and relatively non-intrusive tracking of surgical tools.